In MONICA, the modelling of crowd dynamics from video footage is based on state-of-the-art deep learning techniques within the fields of computer vision and artificial intelligence. A recent demonstration of the MONICA crowd counting algorithm shows a high accuracy of 72 % which is expected to further increase with the labelling of more data.
The MONICA security solution consists of a series of applications which, in combination, can be used to monitor and manage security at large events. Especially CCTV cameras play a central role in the security setup of MONICA, providing the security personnel with important information about crowd size, density and flow. On top of this, the personnel can be notified of potential incidents such as fights or high-risk queues, all of which enables a quick response time and intervention.
Read more about the applications for crowd safety and security
Using IoT for high performance at a low cost
Together with VCA Technology, Kingston University London is supplying the video analytics software which processes and interprets the collected video data. Since commercially available CCTV cameras can only count and track in uncrowded areas due to lack of processing power, the task is to improve the performance so to cover more crowded areas. And this can be achieved by using IoT. As Professor Paolo Remagnino from Kingston University London explains:
‘With the concepts of IoT and cloud computing, we can split the video analytics processing between edge nodes and cloud infrastructure. The additional computer power offered by the cloud and the IoT hybrid processing model we are developing, enable us to create more advanced algorithms which can still use low-cost commercially available sensors but achieve high performance for counting and tracking in crowded scenarios‘.
In 2018, the video analytics was used at most of the MONICA demonstrations for various purposes, including gate counting, crowd size estimation and congestion monitoring. Kingston University London and VCA Technology used the collected data to update the algorithms and prepare for the full video analytics modules.
Performance also relies on deep learning and labelling of data
The first demonstration of the updated algorithms took place on 28th March 2019 at the Emerald Headingley Stadium. The counting algorithm performed well, reaching a counting accuracy of 72 % which is high compared to what is usually possible to achieve. As Paolo Remagnino explains:
‘Modelling crowd dynamics accurately was nearly impossible up until the emerging of deep learning techniques. The new counting algorithm benefits from the state of the art in deep learning which uses artificial neural networks to mimic the structural and functional properties of the biology of the human brain.‘
This artificial intelligence technique makes it possible to achieve a higher accuracy when identifying and counting people from video footage, however, it relies on the presence of meaningful data:
‘Performance of deep learning algorithms depends heavily on the volume and quality of training data supplied to the algorithm. Preparation and annotation of such training data is a challenging and time-consuming task which calls for the collaboration of MONICA pilot organisers. With more labelled data, we expect further enhancements in crowd counting accuracy‘, Paolo Remagnino concludes.
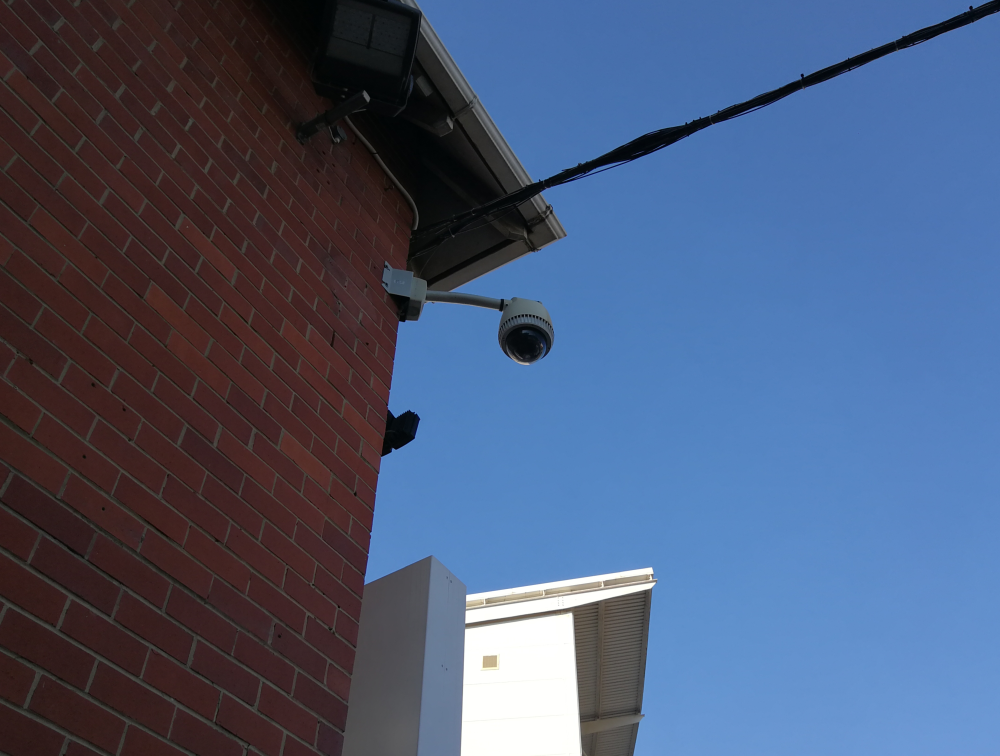
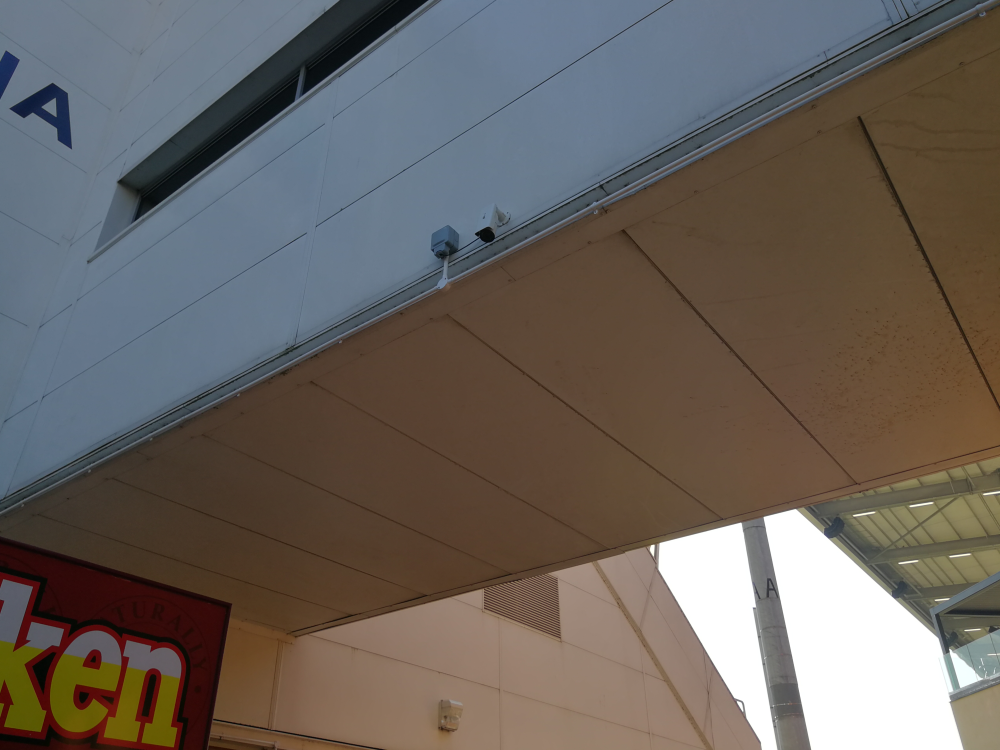
Two cameras were used at a Leeds Rugby match on 28th March 2019 at the Emerald Headingley Stadium. The one on the left is used to estimate crowd size and flow. The one on the right is counting people at one of the gates.
Explore the scientific publications related to crowd and video